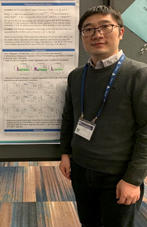
Title: Efficient Algorithms for Nonconvex Sparse Learning Problems
Abstract: Sparse learning plays important roles in the fields of statistical learning, machine learning (ML) and signal processing. Learning with high dimensional data often relies on the sparsity-driven regularization. Solving a sparsity-regularized empirical risk minimization (ERM) problem can derive a ML model with sparse parameters, meaning that the model parameter vector has many zero entries. Thus, it helps select relevant features for use in a ML model. For example, via the sparsity regularization, genomic analysis can identify a small number of genes contributing to the risk of a disease, and smartphone-based healthcare systems can detect the most important mobile health indicators. In this report, sparse learning is formulated as nonconvex and/or nonsmooth optimization problems depending on the specific regularizer. We develop efficient stochastic gradient descent (SGD) based algorithms to solve these problems.
Biography: Guannan Liang obtained his Ph.D. degree at the Computer Science and Engineering, Department of the University of Connecticut in 2021. He received his M.S. degree in Statistics at the University of California, Davis in 2016, and his B.S. degree in Mathematics at Zhengzhou University (China) in 2013. He has published several papers in top-tier machine learning and data mining conference -- Neurips, AAAI, ICDM and CIKM. His primary Ph.D. research focuses on mathematical optimization, scalable machine learning.
Time: 9: 10-10: 10 am, Friday, April 16, 2021
Venue: Room 403, Shui Wu Building
Organizer: School of Artificial Intelligence