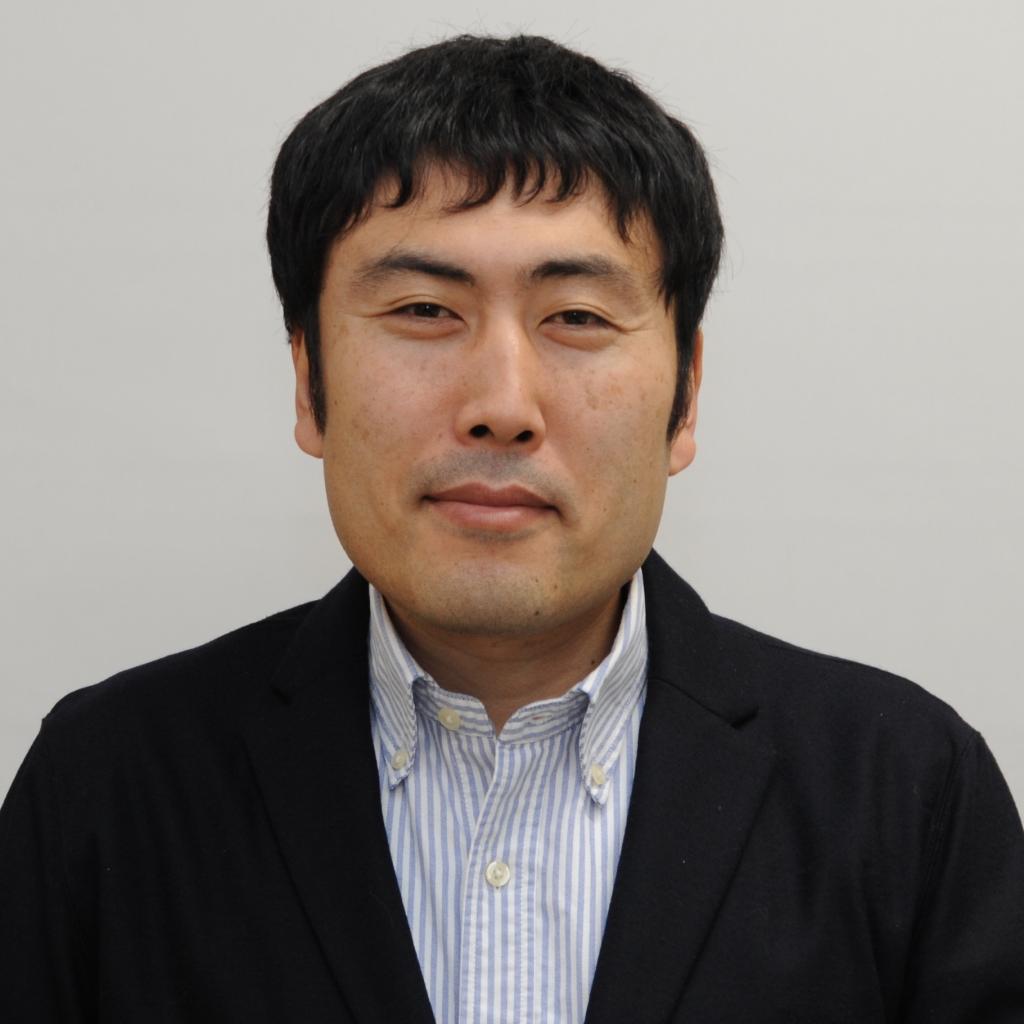
Title: Machine Learning for Sciences — Nonlinear Feature Selection for High-Dimensional Data
Speaker: Makoto Yamada
Time: Monday, October 15, 2018, 09:00-11:00
Lecture Venue: Multi-function Hall, 2nd Floor, Dongrong Convention Center, Central Campus
Organizer: School of Artificial Intelligence, International Center of Future Science
Abstract
Feature selection is an important machine learning problem. However, there are a few methods that can select features from large and ultra high-dimensional data (more than million features) in nonlinear way. In this talk, we first introduce a Hilbert-Schmidt Independence Criterion Lasso (HSIC Lasso) that can efficiently select non-redundant features from a small and high-dimensional data in nonlinear way. A key advantage of HSIC Lasso is that it is a convex method and can find a globally optimal solution. Then we further extend the proposed method to handle ultra high-dimensional data by incorporating with distributed computing framework. Moreover, we introduce two newly proposed algorithms the localized lasso and hsicInf, where the localized lasso is useful for selecting a set of features from each sub-cluster and hsicInf can obtain p-values of selected features from any type of data.
Short biography of Dr. Makoto Yamada
Makoto Yamada, Ph.D., is currently an associate professor at Kyoto University in Japan and the head of the RIKEN AIP. He obtained his master's degree in electrical engineering from Colorado State University in 2005, and a doctorate in statistical science from The Graduate University for Advanced Studies in Japan in 2010. He served as a postdoctoral researcher at the Tokyo Institute of Technology, a researcher at the NTT Communications Science Laboratory, and a research scientist at Yahoo! Reseach. His research interests include machine learning, natural language processing, signal processing, and computer vision. In recent years, he has published more than 30 research papers in top conferences and journals. He won the Best Paper Award in WSDM 2016 and published a paper in Cell in 2018.