The paper ‘Learning English to Chinese Character: Calligraphic Art Production based on Transformer’ of the 2020 postgraduate student YiFan Jin under the guidance of Associate Professor Xi Yang was accepted by the international conference SIGGRAPH Asia′2021 posters. This work was completed in cooperation with Yi Zhang from the National University of Defense Technology.
YiFan Jin came to our school to study for a master's degree in 2020. With much interest in the research of computer graphics, he joined the Interdisciplinary Intelligent Graphics Group (IIGG) and started his research in related fields from the first year. The group hopes everyone ‘enjoy research and have fun’!
Conference Introduction: SIGGRAPH Asia, a computer graphics and interactive technology exhibition and conference organized by ACM SIGGRAPH, is the world's most influential, largest, and authoritative CG as well as interactive technology exhibitions and conferences that integrate science, art, and business. SIGGRAPH Asia will be held in Tokyo Japan as well as online from December 14th to 17th, 2021.
First author: YiFan Jin
Paper topic: Learning English to Chinese Character: Calligraphic Art Production based on Transformer
Conference name: The 14th ACM SIGGRAPH Conference and Exhibition on Computer Graphics and Interactive Techniques in Asia
Conference class: CCF A Conference, Tsinghua Class A Conference
Conference time: 14 – 17 December 2021, Tokyo, Japan
Paper introduction: We propose a transformer-based model to learn Square Word Calligraphy to write English words in the format of a square that resembles Chinese characters. To achieve this task, we compose a dataset by collecting the calligraphic characters created by artist Xu Bing, and label the position of each alphabet in the characters. Taking the input of English alphabets, we introduce a modified transformer-based model to learn the position relationship between each alphabet and predict the transformation parameters for each part to reassemble them as a Chinese character. We show the comparison results between our predicted characters and corresponding characters created by the artist to indicate our proposed model has a good performance on this task, and we also created new characters to show the “creativity" of our model.
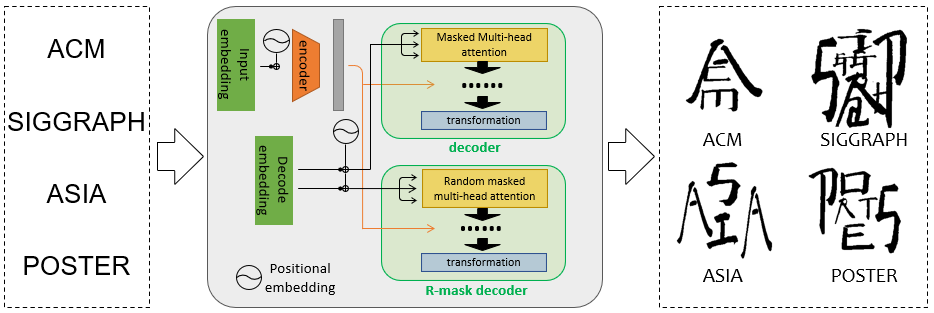